Pay Equity Deep Dive Part III: Tainted Variable Analysis and Root Cause Assessment
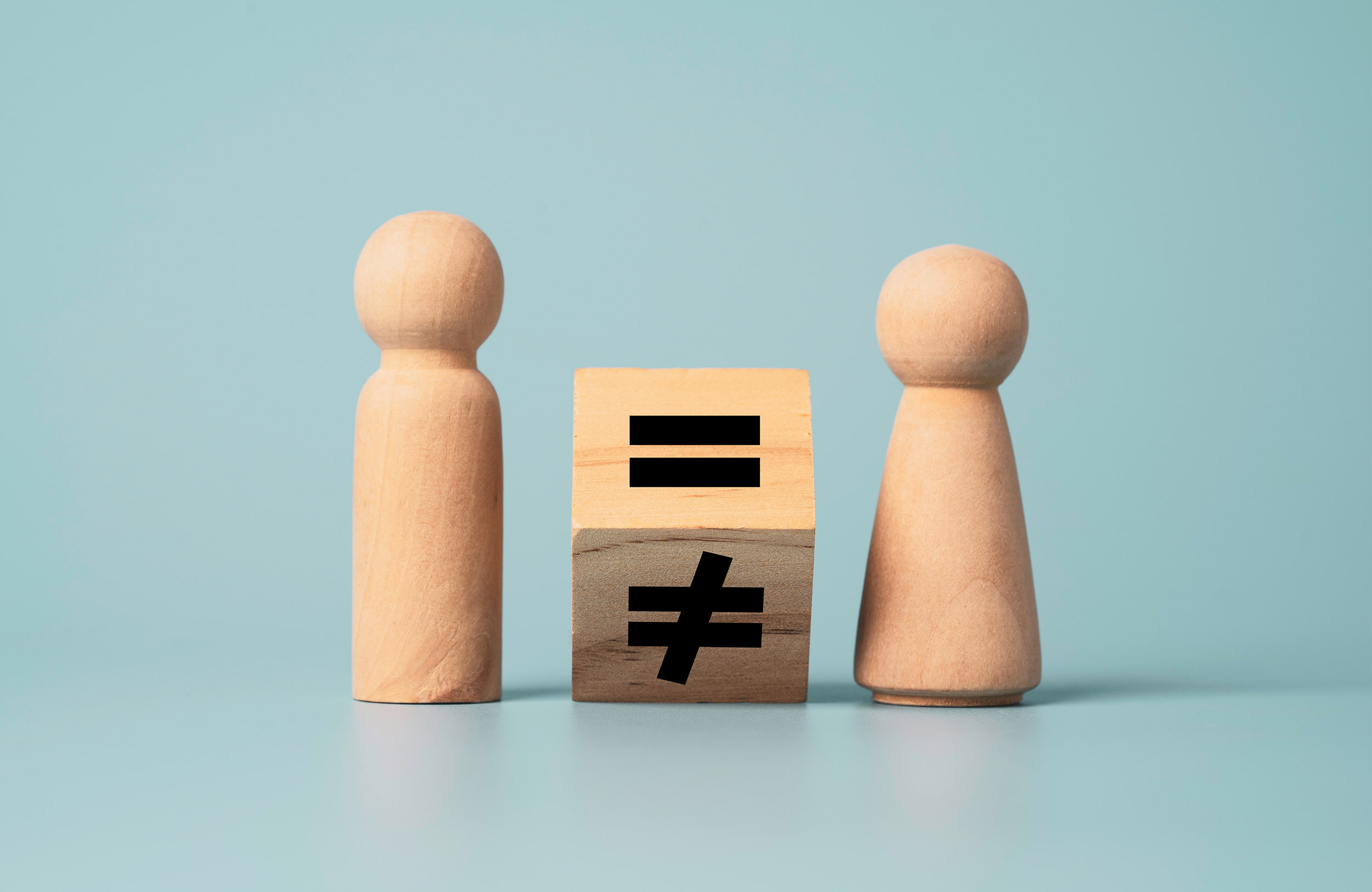
This is Part III of our “Pay Equity Deep Dive Series.” Part I focused on Compensation Philosophy Review and Pay Analysis Group formation and testing. Part II focused on Wage Influencing Factors (WIFs) and Reliability and Robustness Testing. Part III covers Tainted Variable Analysis and Root Cause Assessment.
As we shared in Part II of our deep dive, a Wage Influencing Factor (WIF) is a factor reflecting skill, effort, responsibility, working conditions, or location applied consistently in determining employees’ compensation.
WIFs are meant to be business-related or job-related factors that one would expect to influence employee pay. However, a WIF may both explain much of the raw pay gap and show significant differences related to gender, race/ethnicity, or other protected characteristics. For example, men may be more highly represented in higher career levels, or people of color may receive lower performance reviews. When a WIF has these characteristics, it reflects a feature of the work environment that has a disparate impact on the compensation of employees with different protected characteristics. In such cases, the WIF may be tainted. Tainted WIFs raise concerns about the business necessity of the underlying processes driving these disparate compensation outcomes.
It can be problematic to use a tainted variable as a WIF in a pay model. This is most notably the case for U.S. government contractors who are subject to the requirements of Executive Order 11246. EO 11246, enforced by the EEOC, prohibits qualifying federal contractors from discriminating in employment decisions, including compensation, on the basis of race, color, religion, sex, sexual orientation, gender identity or national origin.
The OFCCP’s 2018 Directive states that in conducting a multiple regression analysis of compensation as part of a compliance evaluation, it will “test all variables for neutrality, and omit any variables that it determines from its evaluation are tainted by discrimination.”
Common Examples of Potentially Tainted WIFs
Any WIF could be statistically related to gender, race/ethnicity, or other protected characteristic. That said, it’s more common among certain WIFs. One example is career level. We regularly include career level as a WIF in a model of compensation. In fact, it’s typically one of the biggest drivers of pay differences among employees.
According to McKinsey’s Women in the Workplace 2023 report, women constitute 48% of entry level employees, 40% of managers, 36% of senior managers/directors, 33% of VPs, 27% of SVPs, and 28% of C-suite employees. This decline in the representation of women as you move up the career hierarchy is a common phenomenon. Thus, it’s common to find that career level is statistically related to gender. We often see something similar when looking at race/ethnicity.
Another example is performance ratings. In 2022, Textio released a study on Language Bias in Performance Feedback. The study analyzed performance feedback for 25,000 people. Here are three of the study’s findings regarding negatively biased performance feedback, defined as personality-based, exaggerated, and non-actionable feedback:
- 41% were women, yet they received 61% of the negatively biased performance feedback language.
- 12% were Latinx, yet they received 29% of the negatively biased performance feedback language.
- 9% were Black, yet they received 32% of the negatively biased performance feedback language.
Other studies have shown a relationship between demographic characteristics and performance ratings. Examples include Potential and the Gender Promotion Gap; A Reexamination of Black–White Mean Differences in Work Performance: More Data, More Moderators; and Ethnic group differences in measures of job performance: A new meta-analysis.
Furthermore, in my experience conducting performance rating bias assessments, the analyses often reveal ratings are statistically related to demographic characteristics.
How to Identify Potentially Tainted WIFs
There isn’t a single correct way to identify if a WIF is potentially tainted — that is, reflects disparate impact across protected groups. Moreover, regulators do not publish their criteria for determining tainted variables. Trusaic, we use five criteria to identify potentially tainted WIFs within a Pay Analysis Group (PAG). These criteria take into account the context in which tainted variables are being evaluated. Specifically, does the WIF vary systematically across different protected classes and would its removal from the analysis reveal a larger and statistically significant pay disparity?
- Raw pay gap between two demographic classes is at least 5%. For example, the average pay of men is at least 5% higher than the average pay of women. Without a practically meaningful raw pay gap, removing a WIF is unlikely to uncover an additional pay disparity.
- Raw pay gap is statistically significant at the 5% level. In addition to being practically meaningful, the raw pay gap is unlikely to be due to chance. If not statistically significant, the raw pay gap may be due to chance.
- WIF accounts for at least 50% of the raw pay gap. For example, career level accounts for 50% or more of the raw pay gap between men and women. Thus, a 5% raw pay gap would be reduced to 2.5% or less by the inclusion of the WIF. This ensures the WIF under consideration is responsible for a sizable portion of the raw pay gap. If the WIF were dropped from the model, it would have a material effect on the pay disparity.
- Distribution for one class across the WIF is significantly different than the distribution for another class. For example, the distribution of women across career levels differs significantly from the distribution of men across career levels.
- WIF stays significantly correlated with class after accounting for other WIFs. For example, the distribution of women across career levels is significantly different from the distribution of men across career levels after accounting for other WIFs included in the pay model (e.g., tenure, time in position).
When assessing whether a WIF is tainted, we give a point for each of the above criteria. A WIF that meets none of the criteria receives a score of zero. A WIF that receives a score of four or five reflects disparate compensation impact and is flagged for review.
What to Do About a Potentially Tainted WIF
When a WIF is flagged as tainted, we recommend reviewing whether the difference is “job-related” and/or “consistent with business necessity.” This might require an investigation of recruiting, retention, performance review, and promotion practices. For example, if performance ratings show evidence of bias, we recommend reviewing the rating process.
The rating process includes all points where decisions are being made (e.g., manager’s initial rating, calibration, final rating). If the rating process shows evidence of bias (e.g., specific managers appear to be rating people based on their demographic characteristics), removing the WIF from the model is advisable.
As another example, if career levels show evidence of bias, examine hire rates, promotion rates, and retention rates. If the examination shows that rates are equitable across demographic classes, then systematic differences in career levels are likely due to other factors, such as talent availability. In this case, the source of the disparate compensation impact is beyond the control of the employer, and the WIF may be included in the model.
What if Drivers of Pay Are Not the Same for All Demographic Classes?
An important part of a pay equity review is developing a model of pay for each Pay Analysis Group (PAG), making sure to follow best practices for WIF consolidation and refinement. As you’ll recall, WIFs are compensable factors that one would expect to influence employee pay. Examples include career level, job function/family, performance rating, company tenure, position tenure, line of business, educational attainment, and geographic location.
As part of conducting a pay equity review, you’ll identify the extent to which WIFs influence pay outcomes. For example, you might find an additional year of time in position increases pay by 1%, while receiving a recent rating of “exceeds expectations” increases pay by 3% relative to someone whose recent rating is “meets expectations.” An underlying assumption is that these “drivers of pay” are the same for all demographic classes.
Following our earlier examples, this means that all demographic classes experience an increase in pay of 1% for each additional year of time in position and a 3% pay premium for exceeding expectations. What if this isn’t the case? What if the drivers of pay differ for different demographic classes?
If the drivers of pay differ for different demographic classes, this could create pay inequities. For example, what if the pay premium for exceeding expectations is 4% for men and 2% for women? This means that women are not rewarded as much for their performance as compared to men. The result of this systematic difference in the way women are rewarded for performance can create a statistical gender pay disparity that will require remedial pay adjustments.
Assuming this systematic difference repeats itself over time, a gender pay disparity will eventually reappear.
Root Cause Assessment
One way to help prevent pay inequities from recurring is to conduct a root cause assessment. The assessment involves analyzing the drivers of pay for different demographic classes (e.g., men vs. women, White vs. BIPOC).
The table below shows an illustrative example of the drivers of pay for men versus women. The first column is the list of WIFs included in the pay model. The second two columns are the drivers of pay for men versus women based on a statistical analysis. For example, the first row of data shows that, all else equal, male managers are paid 15% more than individual contributors, while female managers are paid 16% more than individual contributors. The last column denotes if the difference in the driver value between men and women is statistically significant.
In the illustrative example above, three WIFs show statistically significant differences: VP, Age, and Exceeds Expectations. In all three cases, women are rewarded less for the WIF than men:
- Male VPs are paid 35% more than individual contributors, while female VPs are paid 30% more.
- An additional year of age increases pay by 2% for men and 1% for women.
- A recent rating of “exceeds expectations” (relative to “meets expectations”) increases pay by 4% for men and 2% for women.
Policy Implications
Once you’ve conducted a root cause analysis, the next step is to consider the policy implications for your organization. Looking at the illustrative example above, one possibility for the finding about VP women is that pay increases associated with the move from Director to VP are lower for women than for men. Another possibility is that once at the VP level, merit pay increases are higher for men than for women. Here are two potential policy implications:
- Compensation team reviews promotions from Director to VP to ensure recommended pay increases are in alignment with internal equity.
- Compensation team reviews merit pay recommendations for VPs to ensure recommended merit increases are in alignment with internal equity.
The finding regarding age might require more investigation. In this case, age is used as a proxy for prior work experience and suggests that women are not rewarded for their prior experience as well as men. Using age as a proxy for prior experience may work well for men but less well for women.
A seminal HBR article by Sylvia Ann Hewlett and Carolyn Buck Luce sheds light on the phenomenon of women leaving the workforce at various points in their career. The authors’ survey research found that, “Nearly four in ten highly qualified women (37%) report that they have left work voluntarily at some point in their careers.” Moreover, they found that, “Among women who have children, that statistic rises to 43%.” These research findings suggest that, generally, age may be a less accurate measure of prior work experience for women.
A potential policy implication is to collect data on relevant prior experience for your current workforce and put in place a mechanism to capture this information for new hires. You can then use this more precise measure of prior experience as a WIF in a pay model.
Lastly, women are rewarded less for a rating of “exceeds expectations” as compared to men. One policy implication is to create guidelines for managers that define target merit pay adjustments based on performance rating. Further, create a calibration process to ensure managers are following the guidelines.
* * * * * *
Stay tuned for Part IV of our “Pay Equity Deep-Dive” series, where we’ll discuss developing a remediation strategy.