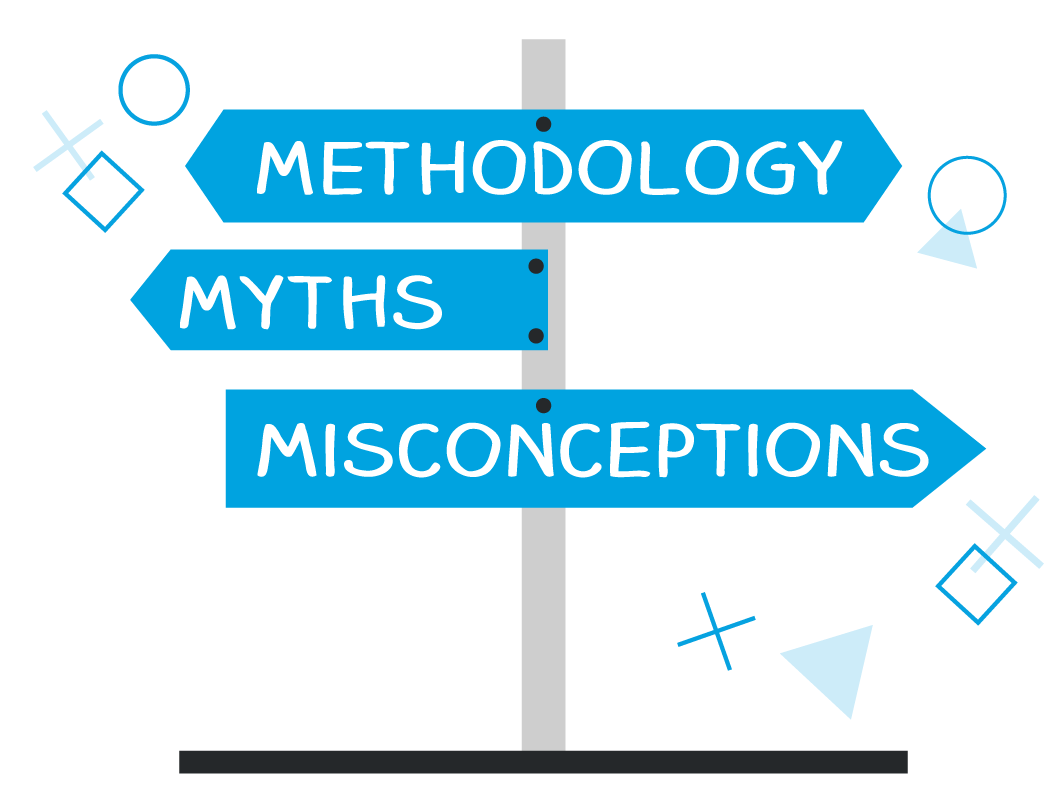
Want more expert insights on the topic of pay equity? Access our entire Pay Equity Deep Dive Series.
Continuing with our Pay Equity Deep Dive Series, we’re turning our attention to pay equity methodology myths and misconceptions. In back-to-back blogs we will identify and examine seven common myths and misconceptions. By sharing and scrutinizing seven common myths and misconceptions, we aim to help organizations refine their methods to address pay equity more effectively.
In the first part of our examination, we focus on myths about analyzing total compensation, segmenting your workforce, factors to include in your pay models, and statistical significance.
Myth 1: If you have a total compensation philosophy, your pay equity analysis should focus on a measure of total compensation (e.g., total cash compensation, total direct compensation).
For organizations with a total compensation philosophy, you may feel compelled to conduct a pay equity analysis on a measure of total compensation (e.g., TCC, TDC). However, there are important caveats to keep in mind.
A pay equity analysis of total compensation is informational, but not directly actionable. The analysis offers no guidance as to which form of compensation (e.g., base, bonus, equity) you should adjust if you discover an inequity in total compensation.
It may be tempting to close an inequity in total compensation by adjusting whichever element of pay is most convenient. We recommend avoiding this tactic, as it can create new inequities and leave existing inequities unaddressed.
For example, if it’s determined that an employee has a $10,000 total compensation inequity that is due to a base pay inequity, closing it with a one-time bonus will result in the continued base pay inequity and may create a bonus inequity.
Optimize Pay Disparity Remediation Efforts With Software
Take particular care with base pay. A base pay inequity has repercussions beyond the amount of base pay an employee takes home. Certain benefits, such as 401(k) match and life insurance, are commonly based on an employee’s base pay. Moreover, targets for other forms of compensation, such as bonuses and equity, are often a percentage of base pay.
If you’d like to examine total compensation, we recommend examining each component of pay individually and remediating each separately.
Myth 2: You should segment your workforce based on job title.
A critical foundational step in conducting a pay equity analysis is segmenting your workforce into Pay Analysis Groups (PAGs). The purpose of segmentation is to group employees into meaningful pools for comparison purposes.
While segmenting by job title may seem like a logical choice, it’s probably not the best approach. Segmenting by job title will likely result in many segments, each with relatively few incumbents.
Under this approach, it’s more likely that a segment will have too few employees to analyze using regression. We do not recommend using non-regression methods in these situations (e.g., Fisher’s Exact test or Mann–Whitney/Wilcoxon rank sum test). Such methods do not allow you to account for relevant Wage Influencing Factors (WIFs), do not provide actionable results, and run the risk of “false negatives” because they have less statistical power than regression.
Moreover, when using regression to analyze small groups, such as job titles, it’s difficult to detect pay inequities because of limited statistical power.
I cannot overstate how important this is.
Small groups may have inequities, but they may not be detected, which obscures risk. For example, women within a specific job level may have a pay disparity of 5% that is not statistically significant when analyzed separately by job title but is statistically significant when pooled and analyzed as a common job level disparity.
Because they often lack statistical power, regression models based on job titles usually are not statistically distinguishable from each other. This means the regression weights associated with the WIFs for a given job title model are not statistically distinguishable from the regression weights associated with the WIFs for another job title model. In such cases, there is no statistical reason not to combine the job titles together into a common job level analysis.
For these reasons, we recommend structuring a pay equity analysis to avoid analyzing small groups. Ultimately, your segmentation needs to balance “differentiation” (which allows you to capture unique pay practices associated with different parts of your workforce) and “pooling” (which improves statistical power).
Myth 3: Your pay models should include as many explanatory factors as possible.
We advise being selective about which Wage Influencing Factors (WIFs) to include in a pay model. The goal is to identify the best collection of factors that explain pay. The following are best practices to consolidate and refine the WIFs included in your models:
- For each categorical WIF in your model, each category value should have at least five employees.
- Be mindful of low-incumbent job titles. For jobs that apply to few employees (e.g., <5), attributes of the job, such as department, division, job level, job family, and location can be used to distinguish compensation levels.
- Aim for a model adjusted R-squared of at least 70%.
- Ensure the ratio of the number of employees in a Pay Analysis Group (PAG) to the number of WIFs is at least five.
- At least 70% of the WIFs in the model should be statistically significant.
Following these best practices will result in more accurate and stable predictions of pay and will reduce the chance of a “false negative” when assessing pay equity risk.
You can find more information on these best practices in a recent blog post.
Myth 4: When identifying a pay disparity, only statistical significance matters.
The statistical significance of a pay disparity is typically measured using a 5% significance level (i.e., p-value ≤ 0.05). This indicates there is a 5% chance or less that the result is due to chance. Statistical significance is the gold standard in pay equity reviews.
That said, there are cases when practical significance should be considered as well. Practical significance indicates whether a pay disparity is meaningful from a business perspective, regardless of its statistical significance.
Under the EU Pay Transparency Directive, disparities exceeding 5% will trigger a joint pay assessment. Thus, in the EU we recommend addressing any disparities above 5%, regardless of statistical significance.
Moreover, it’s conceivable for a company to have no statistically significant disparities in any of its Pay Analysis Groups (PAGs), yet have a statistically significant or practically significant company-wide disparity. To reduce a company-wide disparity, it may be necessary to address PAG-level disparities above a certain threshold (e.g., 2-3%), regardless of statistical significance.
In the next blog of our Pay Equity Deep Dive Series, we will reveal three more common myths and misconceptions. These will dive deeper into your pay equity analysis, examining intersectionality, reference class practices, and remediation.